Resources
Explore libraries, tools, and learning resources for creating stunning audio-reactive visualizations
Essential Libraries
Three.js
A lightweight 3D library that makes WebGL simple. Perfect for creating immersive audio-reactive 3D visualizations in the browser.
Tone.js
A Web Audio framework for creating interactive music in the browser. Essential for professional audio analysis and beat detection.
GSAP
The GreenSock Animation Platform provides professional-grade animation capabilities. Perfect for smooth, performance-optimized transitions.
Theatre.js
A JavaScript motion design library with a visual interface for animation. Great for creating timeline-based audio reactions.
WebMIDI.js
Makes working with MIDI hardware simple. Enables connecting your visualizations directly to MIDI controllers for live performances.
Meyda
Audio feature extraction library for the Web Audio API. Provides advanced audio analysis capabilities beyond basic frequency data.
Code Examples
Basic Audio Analyzer Setup
A foundation for any audio-reactive visualization project. This example initializes the Web Audio API analyzer to extract frequency data from audio.
// Create audio context
const audioContext = new (window.AudioContext || window.webkitAudioContext)();
// Create analyzer node
const analyzer = audioContext.createAnalyser();
analyzer.fftSize = 1024; // Must be power of 2
analyzer.smoothingTimeConstant = 0.85; // 0-1 range
// Frequency data arrays
const frequencyData = new Uint8Array(analyzer.frequencyBinCount);
const timeData = new Uint8Array(analyzer.frequencyBinCount);
// Load and play audio
async function loadAudio(url) {
try {
const response = await fetch(url);
const arrayBuffer = await response.arrayBuffer();
const audioBuffer = await audioContext.decodeAudioData(arrayBuffer);
// Create source node
const source = audioContext.createBufferSource();
source.buffer = audioBuffer;
// Connect audio graph
source.connect(analyzer);
analyzer.connect(audioContext.destination);
// Start playback
source.start(0);
// Begin analysis
requestAnimationFrame(analyzeAudio);
} catch (error) {
console.error('Error loading audio:', error);
}
}
// Analyze audio in animation loop
function analyzeAudio() {
// Get frequency data (0-255 range)
analyzer.getByteFrequencyData(frequencyData);
// Get time domain data (0-255 range)
analyzer.getByteTimeDomainData(timeData);
// Use the data to drive visualizations
updateVisualization(frequencyData, timeData);
// Loop
requestAnimationFrame(analyzeAudio);
}
// Example visualization update function
function updateVisualization(frequencyData, timeData) {
// Calculate average bass energy (frequencies 0-10)
let bassSum = 0;
for (let i = 0; i < 10; i++) {
bassSum += frequencyData[i];
}
const bassAvg = bassSum / 10 / 255; // 0-1 range
// Apply to visualization elements
myVisualElement.scale.set(1 + bassAvg * 2);
}
// Start everything
loadAudio('path/to/audio.mp3');
Audio-Reactive Particle System
Three.js example of particles that react to audio frequency data. Each particle's movement and color are influenced by different frequency bands.
// Create particle system geometry
const particleCount = 5000;
const particles = new THREE.BufferGeometry();
const positions = new Float32Array(particleCount * 3);
const colors = new Float32Array(particleCount * 3);
// Initialize particles in a sphere
for (let i = 0; i < particleCount; i++) {
const i3 = i * 3;
// Random sphere distribution
const radius = Math.random() * 30;
const theta = Math.random() * Math.PI * 2;
const phi = Math.acos(2 * Math.random() - 1);
positions[i3] = radius * Math.sin(phi) * Math.cos(theta);
positions[i3 + 1] = radius * Math.sin(phi) * Math.sin(theta);
positions[i3 + 2] = radius * Math.cos(phi);
// Random initial colors
colors[i3] = Math.random();
colors[i3 + 1] = Math.random();
colors[i3 + 2] = Math.random();
}
particles.setAttribute('position', new THREE.BufferAttribute(positions, 3));
particles.setAttribute('color', new THREE.BufferAttribute(colors, 3));
// Create particle material
const particleMaterial = new THREE.PointsMaterial({
size: 0.5,
vertexColors: true,
transparent: true,
opacity: 0.8
});
// Create particle system
const particleSystem = new THREE.Points(particles, particleMaterial);
scene.add(particleSystem);
// Function to update particles with audio data
function updateParticles(frequencyData) {
const positions = particles.attributes.position.array;
const colors = particles.attributes.color.array;
// Calculate audio energy in different frequency bands
const bassEnergy = getAverageEnergy(frequencyData, 0, 10) / 255;
const midEnergy = getAverageEnergy(frequencyData, 10, 100) / 255;
const highEnergy = getAverageEnergy(frequencyData, 100, 255) / 255;
for (let i = 0; i < particleCount; i++) {
const i3 = i * 3;
// Update positions based on frequency energies
const dist = Math.sqrt(
positions[i3] ** 2 +
positions[i3 + 1] ** 2 +
positions[i3 + 2] ** 2
);
// Different frequency bands affect different dimensions
positions[i3] += Math.sin(dist * 0.1) * bassEnergy * 0.5;
positions[i3 + 1] += Math.cos(dist * 0.05) * midEnergy * 0.3;
positions[i3 + 2] += Math.sin(time * 0.1) * highEnergy * 0.2;
// Update colors based on frequency
colors[i3] = Math.min(1, bassEnergy * 2); // Red - bass
colors[i3 + 1] = Math.min(1, midEnergy * 2); // Green - mids
colors[i3 + 2] = Math.min(1, highEnergy * 2); // Blue - highs
}
particles.attributes.position.needsUpdate = true;
particles.attributes.color.needsUpdate = true;
}
// Helper function to get average energy in a frequency range
function getAverageEnergy(frequencyData, startBin, endBin) {
let sum = 0;
for (let i = startBin; i < endBin; i++) {
sum += frequencyData[i];
}
return sum / (endBin - startBin);
}
Learning Resources
Books & Publications
Online Courses
From Manual to AI: Evolving Your Visual Performance Workflow
How artificial intelligence is revolutionizing audio-reactive visualization creation
The Evolution of Audio Visualization
Audio-reactive visuals have evolved dramatically over the decades, from simple oscilloscope displays to sophisticated real-time 3D environments that respond to every nuance of sound.
While manual programming techniques offer precise control and creative freedom, they come with significant challenges:
- Steep technical learning curve requiring WebGL, shaders, and audio processing expertise
- Time-intensive development cycles that delay creative iteration
- Performance optimization challenges requiring specialized knowledge
The emergence of AI-powered tools like Compeller.ai represents the next evolutionary step, democratizing access to sophisticated audio-reactive visuals.
AI-Powered Benefits
Accelerated Production
Generate in minutes what would take days to code manually
Creative Exploration
Rapidly prototype ideas and explore creative variations
No-Code Solution
Create professional-quality visuals without programming knowledge
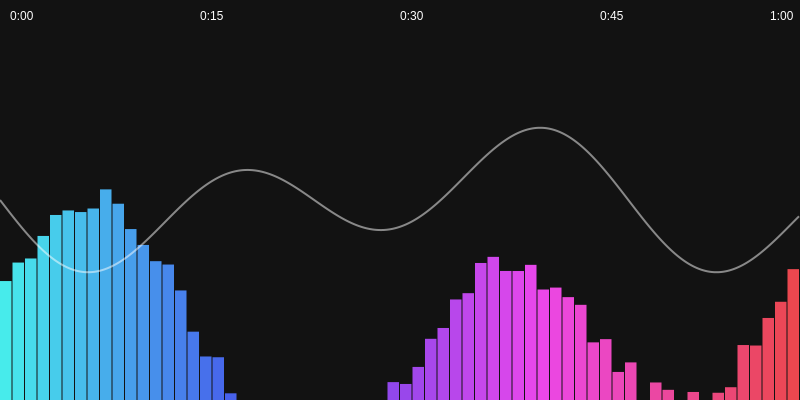
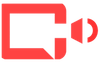
Combining the Best of Both Worlds
The future of audio-reactive visualization isn't about choosing between manual coding or AI—it's about effectively combining both approaches:
Use AI for:
- Rapid prototyping
- Complex pattern generation
- Client presentations
- Deadline-driven projects
Use Manual for:
- Ultra-custom interactions
- Specialized hardware integration
- Precise algorithm control
- Platform-specific optimizations
No credit card required. Forever Free Account.
Stay Updated
Subscribe to our newsletter for the latest tutorials, code examples, and insights on audio-reactive visualization techniques.